What a pickle all of us are in. It has been more than two years since the pandemic hit the world...
The problem with Artificial Intelligence (AI) is the Data, not you
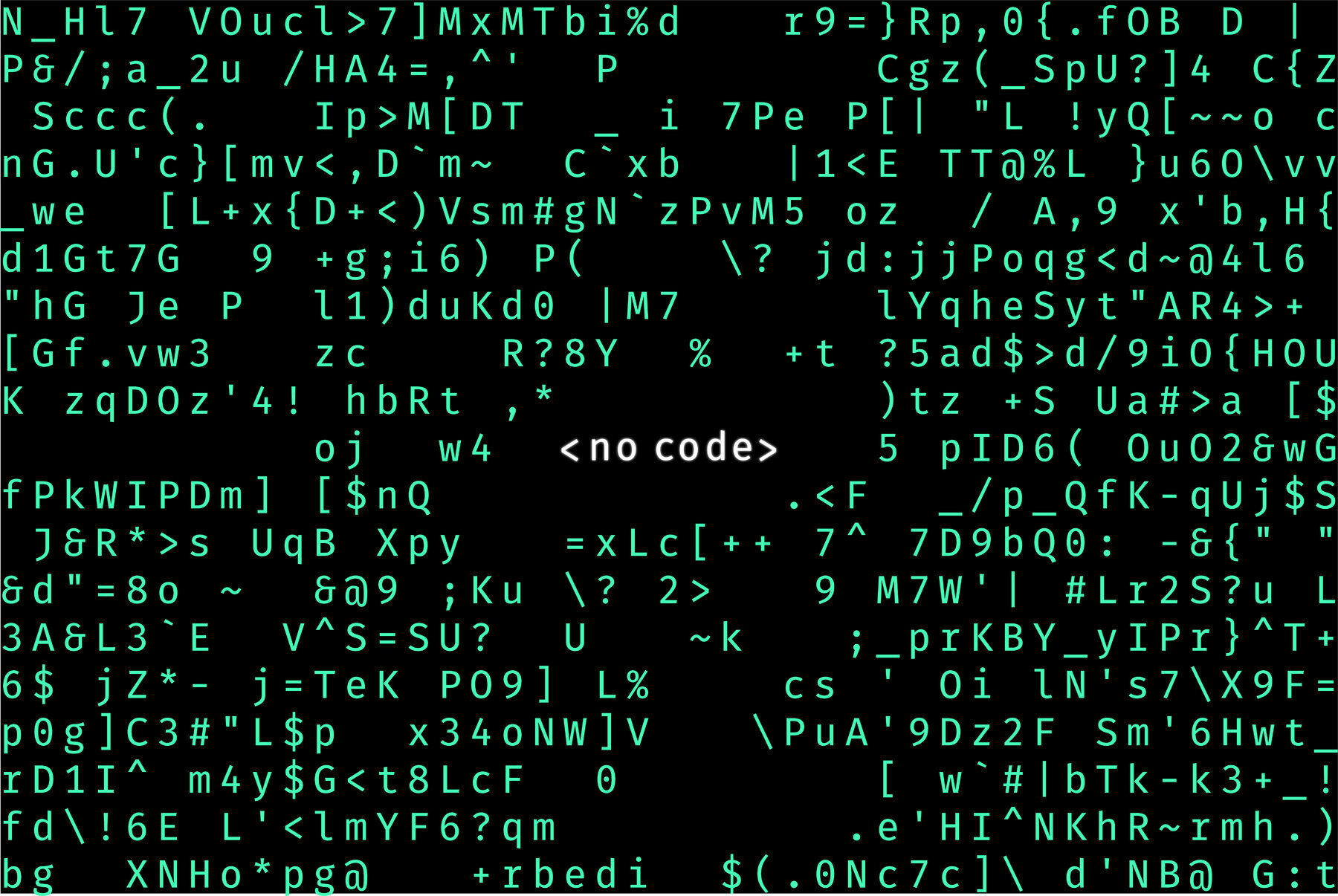
Data should not be viewed as the “new oil,” only meant to be burned once; instead, it should be harnessed for Artificial Intelligence (AI)-driven insights and innovations.
Businesses are throwing away billions because they can’t manage their data effectively. They must better align and support the backend data that feeds these systems if they are to be successful in gaining value through data-driven projects like artificial intelligence.
That’s the main finding of the most recent study, which estimates that businesses could collectively generate more than $460 billion in incremental profit if only people could manage their data resources a little bit better. The study was conducted by Infosys Knowledge Institute and was based on a survey of 2,500 executives.
This includes improving data procedures, having more faith in cutting-edge Artificial Intelligence (AI), and firmly integrating AI with business processes. Value for businesses is still elusive.
The survey identified three obstacles to successful Artificial Intelligence (AI) implementations: a lack of a unified, centralized data strategy; a lack of adequate infrastructure; and a lack of strong data verification. The majority of businesses need a consistent data management strategy.
Although the majority of respondents do not currently do this, they want to manage data centrally. With 26% of respondents presently using a centralized method and 49% hoping to do so by the end of the year, analysis of the survey results “shows that centralized data management links to greater profit and revenue development.
“Data is not the new oil,” the Infosys Institute’s Chad Watt and Jeff Kavanaugh, the study’s authors, place emphasis on this. “Businesses can no longer afford to think of their data as oil, extracted with great effort and valuable only when refined.”
Data today is more like currency: “It gains value when it circulates. Companies that import data and share their own data more extensively achieve better financial results and show greater progress toward ideating AI at enterprise scale — a critical goal for three out of four companies in the survey,” says Watt and Kavanaugh.
The success of currency depends on trust, and the same is true of data. The authors say that trust is necessary for advanced AI. “Trust in your own and others’ data management, and trust in Artificial Intelligence (AI) models. New data and perfectly programmed AI models mean nothing if humans do not trust and use what data and AI produce.”
According to the poll, organizations that shared data within and outside of their organization were more likely to generate more sales and use AI more successfully. “Refreshing data closer to real-time also correlates with increased profits and revenue.”
The study’s authors also suggested that data is more similar to nuclear power than fossil fuel. “Data is enriched with potential, in need of special handling, and dangerous if you lose control. Twenty-first-century data has a long half-life. When to use it, where to use it, and how to control it are as critical as where to put it.”
According to the survey, the majority of firms are still learning about AI. Only 81% of businesses, or more than 8 out of 10, have implemented their first real AI system in the recent four years, and 50% in the last two. In addition, 63% of AI models are controlled by humans and only have basic functions. They keep falling short in terms of data practices, data strategies, and data verification. Practitioners’ satisfaction with their data and AI tools is only at 26% level. The authors of the survey believe that “something is plainly lacking” despite AI’s attraction.
The authors of the survey identified high-performing businesses, which typically place a lot of importance on three things:
- They transform data management into data sharing. “Companies that embrace the data-sharing economy generate greater value from their data,” says Watt and Kavanaugh. “Data increases in value when treated like currency and circulated through hub-and-spoke data management models. Companies that refresh data with low latency generate more profit, revenue, and subjective measures of value.”
- They have made the move from data compliance to data trust. “Companies highly satisfied with their AI (currently only 21%) have consistently trustworthy, ethical, and responsible data practices. These prerequisites tackle challenges of data verification and bias, build trust, and enable practitioners to use deep learning and other advanced algorithms.”
- They engage everyone in the AI process. “Extend the AI team beyond data scientists. Businesses that apply data science to practical requirements create value. Business leaders matter as much as data scientists. Good AI teams typically involve multiple disciplines. “Data verification is the greatest challenge to moving forward, along with AI infrastructure and compute resources.
Here at CourseMonster, we know how hard it may be to find the right time and funds for training. We provide effective training programs that enable you to select the training option that best meets the demands of your company.
For more information, please get in touch with one of our course advisers today or contact us at training@coursemonster.com