When productivity and cooperation are strained, machine learning models can’t be audited or...
Introducing the Process of Data Mining that Ensure Effective AI
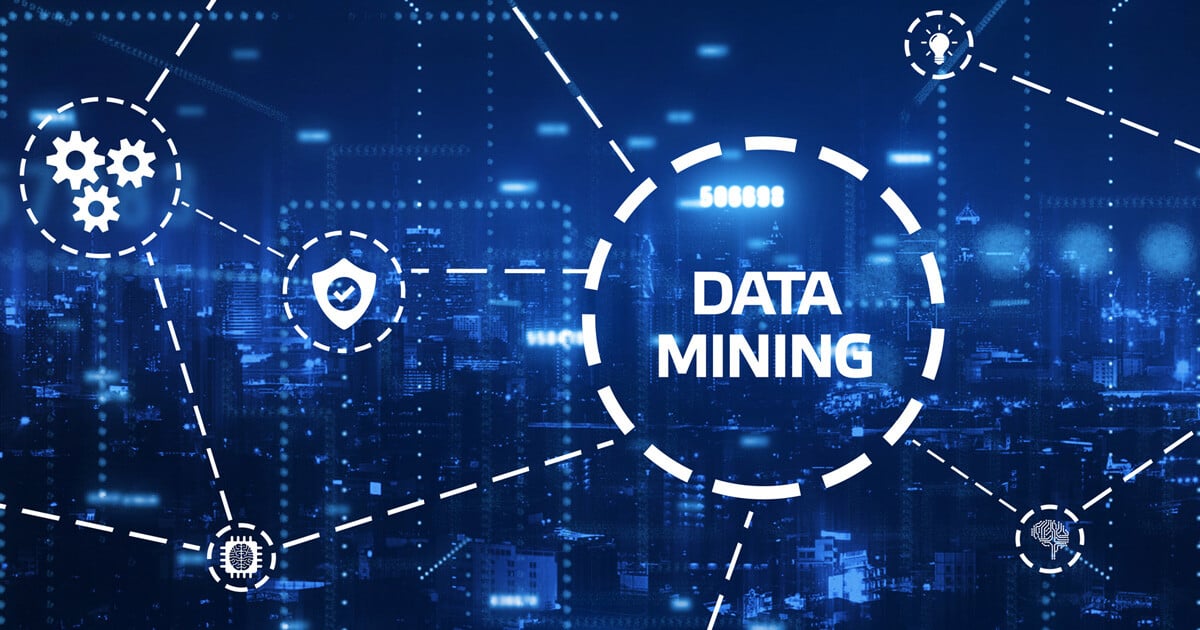
Data, like data mining says that scientists can unintentionally introduce human bias into their models since we are sometimes so driven to create the ideal model. Usually, bias is introduced through training data, increased, and then encoded in the model. Such a model may have major consequences if it is put into production, such as inaccurate credit scores or health assessment predictions. Regulatory standards for model fairness and reliable AI are intended to stop biased models from entering production cycles across a variety of industries.
When creating a model pipeline, a good data scientist must take into account two important factors:
- Bias: People of different groups (or races, genders, ethnic groups, etc.) are usually biased against a model that generates predictions for them.
- Unfairness: a model that predicts in ways that steal individuals of their property or rights without their knowledge
It might be difficult to recognize and define bias and unfairness. Data risk assessment, model risk assessment, and production monitoring should be added as additional elements to the conventional data mining process to help data scientists in reflecting on and identify potential ethical concerns.
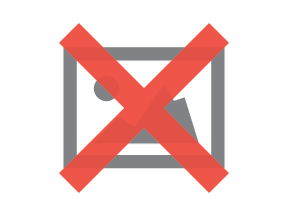
1. Data risk assessment
A data scientist like data mining can analyze any imbalances between various groups of people and the goal variable in this step. For instance, we continue to see that men are still more frequently accepted for managerial positions than women. However, since it is unlawful to discriminate against applicants for jobs based on their gender, you could counter that gender is irrelevant and should be eliminated in order to balance the model. But what other effects might the removal of gender have? To determine whether the present checks are sufficient to limit potential bias in the model, this step should be discussed with the appropriate specialists before being taken.
To guarantee that the training data mining is as similar as feasible to the data used in real-time in the production environment, the purpose of data balancing is to mimic the distribution of data used in the production. Therefore, even if the first instinct is to eliminate the biased variable, this course of action is unlikely to provide a solution. Variables are usually correlated, and bias might enter the model by hiding in one of the associated fields and acting as a substitute proxy. To make sure the bias is genuinely gone, all associations should be checked before being removed.
2. Model risk management
Model forecasts have immediate and significant ramifications; in fact, they have the power to completely alter someone’s life. Your life may be negatively impacted if a model predicts that you have a poor credit score since you may find it difficult to obtain credit cards and loans, find housing, or obtain affordable interest rates. Additionally, if you don’t learn why you got a bad grade, you have little chance of improving.
A data scientist’s or data mining is responsibility is to make sure a model produces the fairest results for everyone. If the data are biased, the model will take that bias into account and produce inaccurate predictions. Black-box models produce excellent results, but because they are difficult to comprehend and understand, it is impossible to look for red signs that might indicate unfairness. Therefore, a thorough examination of model results is required. Data scientists must evaluate the trade-off between interpretability and model performance and choose models that best meet both demands.
3. Production monitoring
Data scientists usually submit their finished models to the MLOps team. When the new model data is used, it may introduce a new possibility for bias or increase the bias that was previously ignored due to a lack of effective supervision. Production data might introduce bias into the model and data and cause performance or consistency to veer off course. Using a tool like IBM Watson Studio, it’s important to manage models by implementing appropriate alarms signaling deterioration of model performance and a system for determining when to retire a model that’s no longer fit for usage. Once more, data quality should be monitored by contrasting the distribution of production data with the data used to train the model.
Want to know more about IBM Watson? Visit our course now.
Responsible data science involves thinking beyond the code and performance of the model, and it is greatly influenced by the data you are using and how reliable it is. In the end, bias prevention is a challenging but essential procedure that ensures that models imitate the proper human processes. This doesn’t imply that you should take any new actions, but it is vital to reconsider and reframe the work that data scientists already undertake to make sure that it is done in a responsible manner.
Get our exclusive guide about the Introduction of Process of Data Mining. Visit us here.
Here at CourseMonster, we know how hard it may be to find the right time and funds for training. We provide effective training programs that enable you to select the training option that best meets the demands of your company.
For more information, please get in touch with one of our course advisers today or contact us at training@coursemonster.com